Optimize Your IT Systems
IT Services & Solutions
Sit back and relax with QSOL IT's full suite of IT Services and Solutions
Professional Services
Architecture, Audit, and Implementation Services to ensure you meet or exceed industry standards.
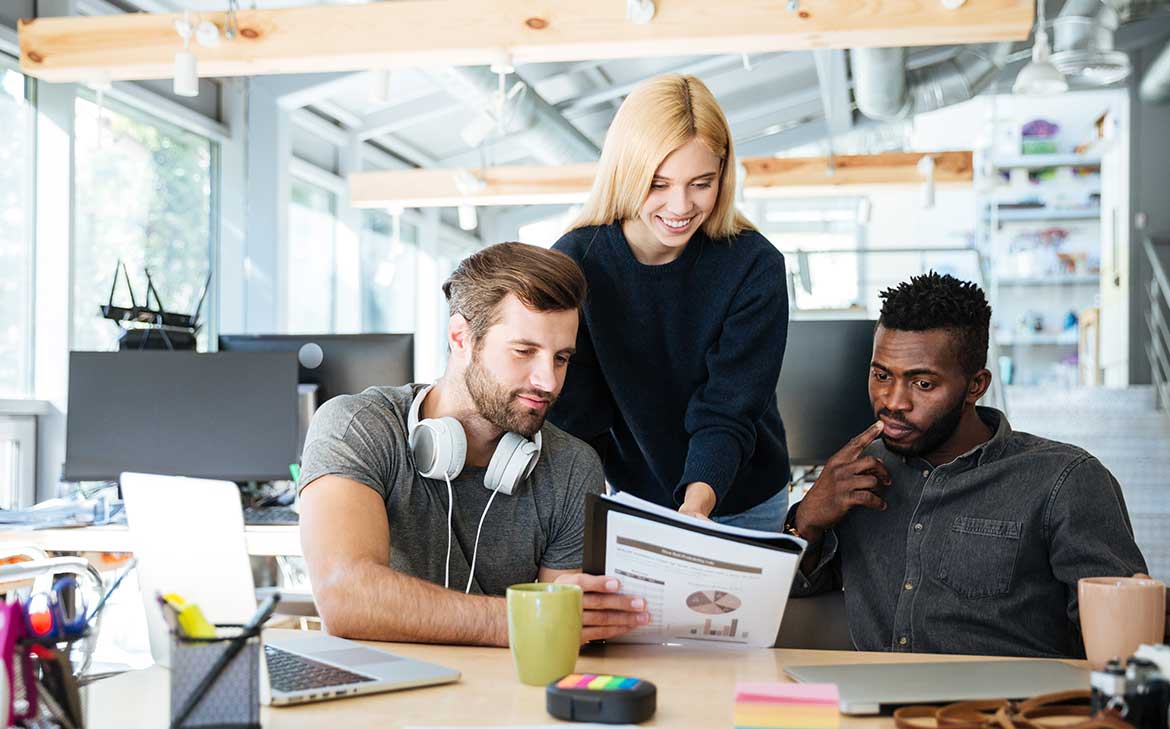
YOUR IT MATTERS
Your Business Matters &
We're Here to Make your IT Easy
Voice and Data Systems are crucial to the success or failure of most businesses. QSOL IT constantly works to ensure your IT Environment helps drive your business towards your vision of success.
24x7x365 Support
QSOL IT's World Class Support is available to you 24x7x365. You are never alone when it comes to your IT. Just open a ticket and watch any worries float away.
Hands Free Implementations
Using Proprietary & Industry Leading Systems, QSOL IT makes any implementation a breeze. You dictate what you want and we will make it happen.
Our Specialized IT Services
We are Preparing for your Success,
By Providing IT Solutions Designed Just For You.
System Certification and Management
Your systems are fundamental to your business. QSOL IT will ensure they are always secure, running and peak performance, and ready for you to get the job done.
Rapid Response Support
24x7x365 Rapid Response White Glove Support ensures if something goes wrong, it's fixed before you even finished your coffee.
Hardware Procurement & Services
Via Partnerships with the leading manufacturers, QSOL IT can easily procure, configure & deploy hardware of your choice and prices that can't be beat.
XaaS
QSOL IT's Anything as a Service (XaaS) model ensures you never need to worry when it comes to your IT. If you want it, we've got it, guaranteed.
Try us, "we can't do it" isn't in our vocabulary.
Try us, "we can't do it" isn't in our vocabulary.
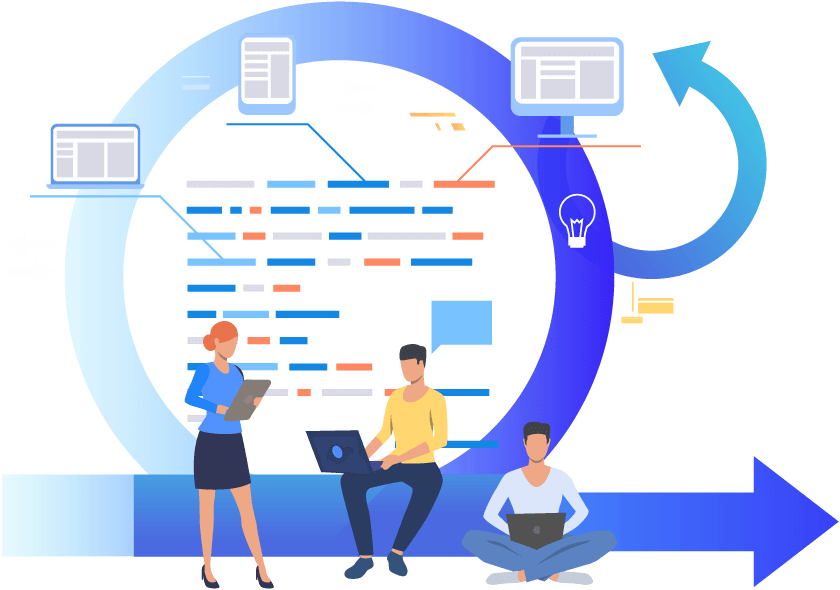